With the incredible amount of coverage and noise ChatGPT has created recently, it’s a sure bet you’re hearing more and more buzz about Artificial Intelligence (AI) than ever before. For years, industries as diverse as financial services, healthcare, manufacturing, and other verticals have embraced machine learning to identify patterns in vast customer, revenue, and production data sets, making quick work of a tasks often ill-suited for humans. Traditionally, AI has applied these “machine learnings’ to help their advisors and sales teams identify “next best actions” to accelerate growth, product adoption, and profitability.
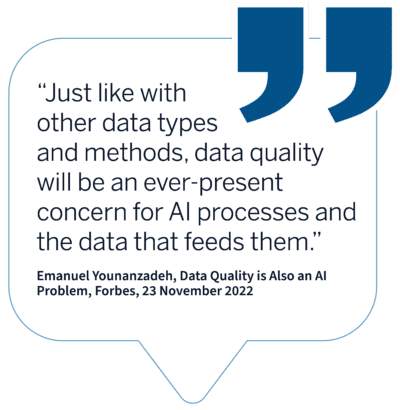
For illustrative purposes, it’s useful—and entertaining—to consider the current hype around consumer-oriented, AI-driven writing technologies like ChatGPT. Currently, in its fourth generation (at time of writing), the technology isn’t exactly new. ChatGPT has elevated its exposure and global impact as everyone from students to speechwriters, explores its capabilities. And those capabilities cover a lot of ground. ChatGPT can write papers. It can plan vacation itineraries. It can write code. It can create apps. It can even accumulate seemingly comprehensive individual dossiers. And while these capabilities can range from impressive to unsettling (will AI take my job? Will it misrepresent me to my potential employers?), a closer look at AI outputs quickly reveals one of its critical vulnerabilities: ChatGPT and other forms of AI are only as good as the data used to train them!
When you consider how AI works, its dependency on accurate and truthful data is immediately apparent. Large Language Models (LLM) draw on the data you provide and on the resources they are told to consult. So, when you “talk to” an LLM like ChatGPT where you don’t control the data it’s going to consult, its output is based on the information and updates it receives to make probability-based predictions.
One of the great hazards of an innovation like ChatGPT is that it’s good at providing compelling responses—even when they’re wrong. Many of us have read of or experienced cases where ChatGPT’s logic arrives at a partially or entirely misleading conclusion. And why does this occur? Because LLM model outputs are based on inaccurate, incomplete, and outdated data. And because ChatGPT is very good at supporting its conclusions in a clear, articulate way, it’s tempting to take its output at face value without questioning whether the output is true.
These concerns also apply to using LLMs to improve sales enablement and “next best action” recommendations. Without a revenue data operations integration solution that is highly focused on data quality and relevance – like Riva, companies run the risk that AI outputs won’t reflect accurate customer histories, interaction insights, or pattern predictions. As a result, suggested next actions can not only be wrong, they can even be harmful to an existing long-term relationship.
Bad Data on Steroids: How AI Can Go Wrong
AI isn’t new for people who work in enterprise environments. Its origins trace back to the 1950s when researchers began exploring the idea that computers could be programmed to find patterns in large data sources—and use those patterns to make predictions. While research on this subset of AI, known as Machine Learning (ML) continued for decades, it wasn’t until recently that its use in corporate settings began to grow exponentially.
Because machine learning was—and is—reliant on raw data (primarily statistics, words, and images), its predictive capabilities rely on the quality, accuracy and relevancy of its data sources. As a result, inaccurate, incomplete, and irrelevant data sets produce predictions that are inaccurate and incomplete, which means:
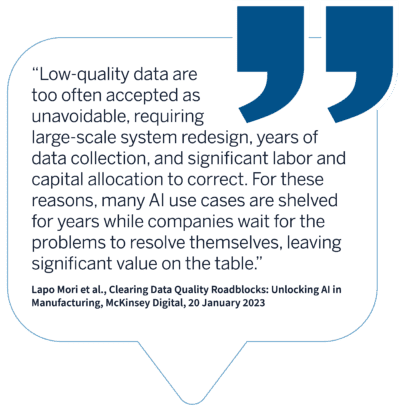
AI can get things wrong
If your machine learning tools rely on data sets that are inherently wrong, the conclusions AI reaches will be wrong too. For example, AI outputs that are generated from siloed systems (think Salesforce and other leading CRMs) can produce inaccurate or incomplete customer histories, and conflicting data sources. How often do customer-facing staff and executives encounter CRM revenue data that says one thing, and emails and interactions from customers that say something else—all of which leads to misguided conclusions and actions.
AI can create conflicts between customer-facing teams and their managers
Given their direct involvement, customer-facing teams have a clear understanding of their direct interactions with enterprise customers. But when AI relies on an incomplete or inaccurate data set that is based solely on revenue data—and managers embrace it—they may be at odds with go-to-market team conclusions about how to best serve customer needs.
AI amplifies the shortcomings of CRM-native data connectors
Experience has shown that native data connectors (e.g., Salesforce Einstein Activity Capture) often capture some—but not all relevant—and often too much customer data just gets amplified into noise.
AI carries forth the biases of its data sources
If machine learning draws on subjective and limited data, its predictions will be tainted by confirmation bias—leading enterprise teams to make or advocate for decisions that might perpetuate misguided recommendations.
AI can be used to achieve nefarious ends
Ill-intended humans can game the data sources relied on by machine learning tools to inform conclusions that may actually work against enterprise objectives.
Transformative Potential: How AI can Use Good Data to get it Right
Over the past decade, enterprises have increasingly—and rapidly—turned to AI to automate a range of tasks traditionally carried out by humans. This trend is seen first and foremost in and has been particularly pronounced in customer engagement, where AI has proven enormously useful for its ability to process vast volumes of revenue and communications data and identify opportunities to leverage that data to build customer relationships and grow customer lifetime value.
When carefully implemented and informed by accurate, complete, high-quality, relevant, and qualified data, AI’s impacts have been profound. They have delivered positive impacts on enterprise companies’ ability to accelerate product and service development, anticipate customer needs, and even enhance customer experiences.
Key areas where AI’s capabilities have successfully and effectively leveraged high-quality data include:
- Process automation;
- Cognitive insight;
- Cognitive engagement.
Let’s look at these areas in more details.
1. Process automation
Automation of administrative tasks—like transferring email data to CRM, reconciling billing errors, or extracting provisions from contract documents—have resulted in dramatic efficiency increases, error reduction, and reduced costs.
2. Cognitive insight
One of AI’s primary advantages is its ability to process vast volumes of data and recognize business-critical patterns. When that data is based on high-quality and relevant data, AI’s pattern recognition capabilities can be used to predict customer behavior, identify fraud, automate ad targeting, and identify product and process defects and safety issues.
3. Cognitive engagement
With AI, automated customer service has gone from being a novel idea to becoming ubiquitous in a few short years. Systems that use natural language and predictive technologies to hone in on (hopefully) relevant solutions aren’t limited to helpdesks. They are often the first step used across many business units to make product recommendations, help employees troubleshoot technology issues, diagnose and triage healthcare, and even to increase customer engagement personalization.
How to AI-optimize Your Data Quality
When you make the commitment to embrace AI’s positive potential, recognizing its dependence on high-quality data is the most important first step. But if the commitment and tools necessary to produce high-quality data are not applied from the start, AI is more likely to harm your enterprise than to advance its objectives.
Fortunately, revenue data operations solutions like Riva can have an immediate and profound impact on efforts to improve data quality at every interaction phase—and support successful AI initiatives. With revenue data operations integration, enterprise data quality improves through:
1. Unified revenue and communications data
Revenue data operations are designed to eliminate siloing that separates revenue and communications data. This unification expands the universe of data available to your AI technologies to ensure its conclusions are based on current, comprehensive, relevant, and accurate customer histories.
2. Sophisticated data access and use governance
Once data is classified, gathered, and unified, revenue data operations work to govern data flows. This process makes it possible to refine and curate relevant data for use and analysis by AI.
3. Improved data observability
Revenue data operations solutions like Riva increase data visibility at every point in its lifespan. This elevated level of observability ensures that errors, omissions, and duplications are identified and resolved before they can be consumed—and propagated—by your AI technologies.
4. Improved “single source of truth” adoption
When customer-facing teams understand how important it is for them to have and be able to rely on good data, they’re more inclined to embrace their roles and contribute as good data stewards. This fuels a virtuous cycle that sustains data quality—thus enhancing the value and performance of the AI technologies it fuels.
5. Reduced data security and compliance concerns
Revenue data operations solutions like Riva are designed to enforce data use compliance and security. This safeguard minimizes the risk that AI could inadvertently (or intentionally) become responsible or magnify the threat of data non-compliance or data breaches in regulated industries.
Conclusion
Given its rapid rise and accelerating adoption as a data technology enabler, the use of AI to leverage the power of data has quickly become an enterprise inevitability. And while it’s now more essential than ever, improving data quality won’t happen without a commitment by individual contributors and as part of a larger corporate focus. Fortunately, implementing a proven revenue data operations solution like Riva can go a long way toward achieving your commitment to unify and govern the accuracy, consistency, and relevance of the data your AI tools need to help, not harm, your enterprise goals.
Recent Articles
- Guide to Einstein Activity Capture: All You Need to Know
- 2024 Bankers Summit: 5 Key Takeaways
- Financial Services: Mastering Customer 360 and Client Engagement for Increased Customer Lifetime Value
- Dreaming Big at Salesforce Education Summit San Diego 2024
- How to Build a Sales Cadence in 2024: Examples and Best Practices